
https://arcprize.org/competition
>=85% performance on Chollet's abstraction and reasoning corpus, private set.
(If Chollet et al. change the requirements for the grand prize in 2025, this question will not change. The bar will remain >=85% performance)
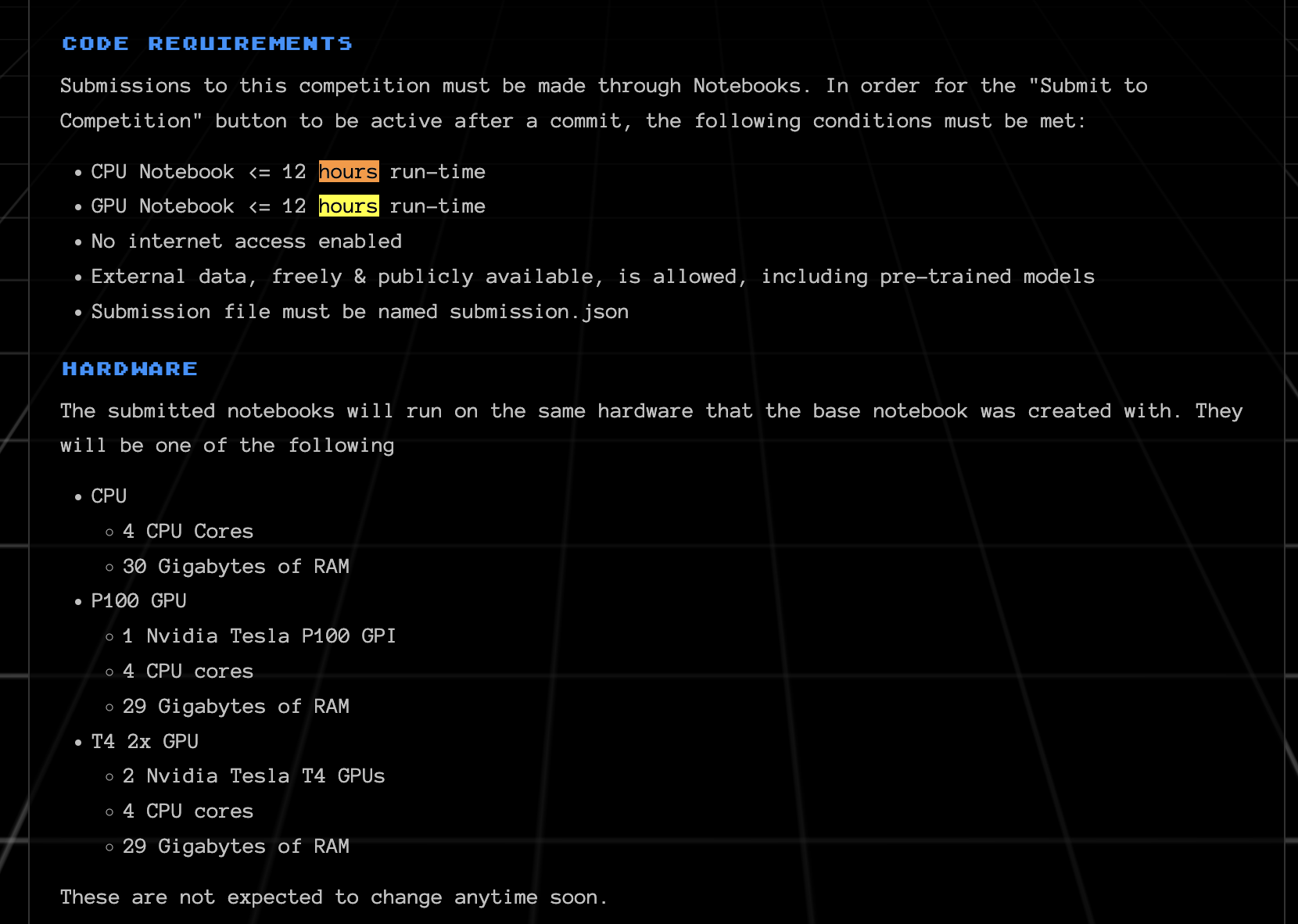
Update 2024-20-12 (PST) (AI summary of creator comment): - This market uses the grand prize rules from ARC-AGI, not the public prize rules
The 87.5% score mentioned in comments was for the semi-private dataset, which does not satisfy the grand prize criteria requiring performance on the private dataset
Update 2024-20-12 (PST): - Market will be resolved based on the original 2024 ARC-AGI test set ("ARC-AGI-1"), not the updated ARC-AGI-2 dataset (AI summary of creator comment)
I'm confused by the difference between this market and this one:
https://manifold.markets/yaqubali/will-the-arc-agi-grand-prize-be-cla-khaivmwh6j
@mathwizurd For the market you linked, it is clear from both title and description that the grand price needs to be claimed for it to resolve YES. To claim the price, >=85% on the updated private test set need to be achieved.
The same should obviously be true for this market here, but the creator now decided that they don't care about the price, but rather about the old/original test set.
For the record, in evaluating any claims made about market resolution: please note who is incentivized to be careful and fair regarding resolution of this question/is speaking in good faith here.
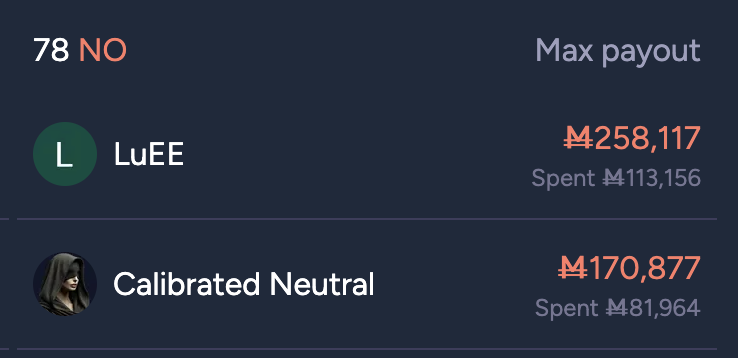
Also note the original question text: "(If Chollet et al. change the requirements for the grand prize in 2025, this question will not change. The bar will remain >=85% performance)"
Given this text, would you assume the dataset would remain fixed (v1), or we would change to a new dataset having an entirely different difficulty level (v2)?
(I'm wasting too much time responding to one person's attempt to skew outcomes in their favor, so I'm going to block Calibrated Neutral. If this person raises any later valid concerns about this market I'm happy to respond if someone else copies the concern and tags me)
The competition next year will run on ARC-AGI-2, an updated version of the dataset that keeps the same format as v1, but features fewer tasks that can be easily brute-forced. Early indications are that ARC-AGI-v2 will represent a complete reset of the state-of-the-art, and it will remain extremely difficult for o3. Meanwhile, a smart human or a small panel of average humans would still be able to score >95%.
@JacobPfau Will you resolve this market based on results on the original test set, "ARC-AGI-1"? (That seems to follow from your description.) I guess they'll still report that too.
@JacobPfau This market is explicitly named 'Will the ARC-AGI grand price be claimed by end of 2025?'. To win the price one needs to reach 85% on the updated private set.
(If Chollet et al. change the requirements for the grand prize in 2025, this question will not change. The bar will remain >=85% performance)
This indicates it only needs 85%+ on the previous dataset I'm pretty sure
@Bayesian The relevant achievement is getting >=85% on the private benchmark. If Chollet et al. had changed the requirements to requiring only 60% on the private benchmark, because the original target turned out to be too hard and they wanted to allocate the price anyway, then this market should have still been resolved YES once 85% are hit due to the sentence you quoted.
The private benchmark is supposed to be private. Information leaks from it every time the creators report the score a model achieved on it. It makes sense to continually update it as long as the newly added tasks are true to the spirit of ARC. The private benchmark stays the private benchmark.
@CalibratedNeutral Oh, my understanding was that the new questions added are meant to be different from the previous ones, to be questions that even o3 doesn't succeed at, but humans still succeed at. If that's wrong and they're along the same distribution but trying to deal with data leaks it's less of an important difference ig. but yeah we agree, if he dropped requirement to 60% that wouldn't matter for this market
@Bayesian It is a tricky question what it means for tasks to be from the same distribution, since they are handcrafted in the case of ARC, as opposed to, say, photographs of natural objects in the wild. I think that it is pretty clear though (and has been mentioned by Chollet many times before) that leakage is bound to occur here, and that one can easily add synthetic training data by having people create new samples from the known heuristics that were used to create the ARC datasets (and come up with similar ones).
Since OpenAI obviously hugely benefits from reporting large score improvements on (at least the semi-private) ARC test set, nobody can tell what kind of resources (in terms of newly created training data, let alone money to train the models) they poured into improving performance on this benchmark already. Obviously, neither that nor ensembling many Kaggle models to artificially increase the score is in the spirit of ARC.
Combining that with the fact that this market's title specifically references winning the grand prize, I feel scammed by the addendum to the resolution criteria.
@JacobPfau it would be great if you could address this.
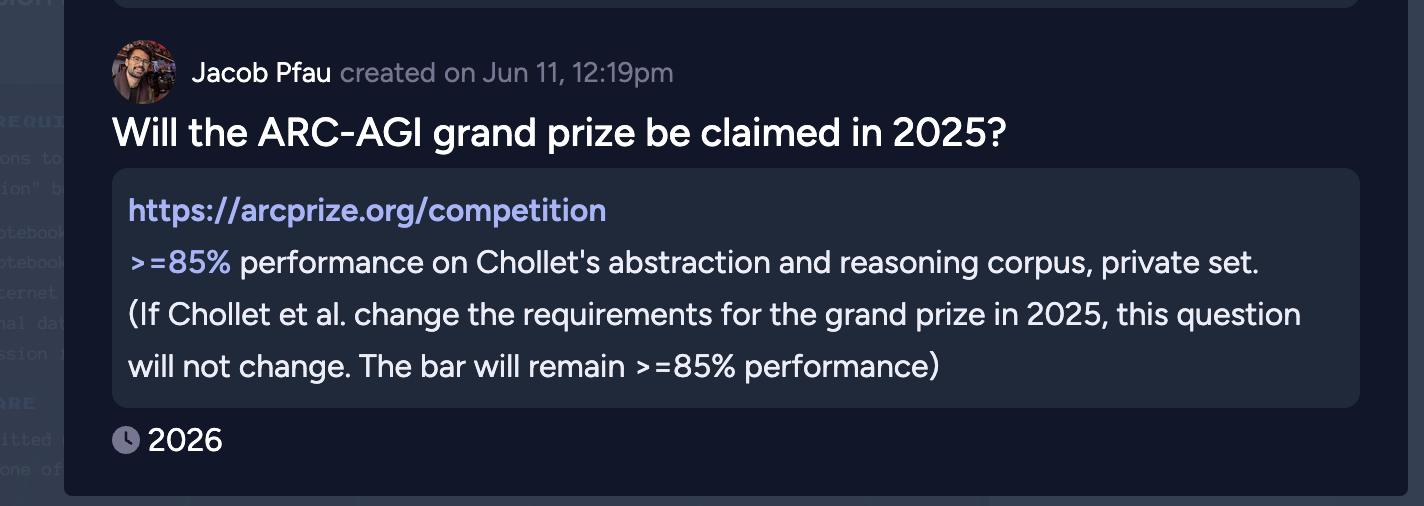
TBC this clarifying text was the second line of the question since inception of this question. I am aware of Chollet-types moving goalposts annually and did not want my question undermined by this.
Concern about gradual leakage strikes me as plausible, but the point of predictions markets is to fix one version of an event and then predict on that. Unfortunately fully specifying things in the title isn't possible usually (perhaps this would've been better as '2024-defined grand prize', but that's beyond the standard of precision for Manifold question titles IME. FWIW, my largest losses on Manifold are because of misleading titles so I sympathize with this, but in this case I think my title + line 2 are relatively clear on what's meant here.
For the record I do not hold any position in this market.
@JacobPfau Changing the title to align with your interpretation is overdue then, and arguably it should have been "Will >=85% be reached on the current* version of the ARC private set by end of 2025" (*this can read "2024" now that an update happened) from the start. Claiming the grand prize is claiming the grand prize, and nothing else. The title as it is now is not merely not fully specifying things but actively misleading.
I read your line 2 as saying that if the resolution criteria change (e.g. the percentage that needs to be reached on the private benchmark becomes either higher or lower, or the compute requirements go up or down), then you would adhere to the original criteria. These criteria are unchanged though: 85% on the private test set, which is conceptually the same as claiming the grand prize (otherwise, why did you title the market like this?).
@JacobPfau Just to clarify: If someone breaks into Chollet's apartment, steals the USB stick containing the original private test set, creates a deterministic algorithm that maps inputs to outputs, and achieves 100% within the compute limit, you will resolve this market to YES, as that scenario aligns with the market spirit you intended, correct?
I assume any reasonable observer, particularly one with ML domain knowledge, would in such a case expect the private test set to be recreated by sampling new data points from the underlying distribution. However, that does not change the fact that the goal is to achieve >=85% performance on samples from that distribution.
I still completely disagree with your interpretation of line 2, especially when read alongside the title, "Will the ARC-AGI grand prize be claimed by the end of 2025?"
@CalibratedNeutral hey, do you wanna bet on what the discrepancy between the 2024 dataset and the 2025 revised dataset will be, in terms of difficulty? Like I'd bet the average AI model does at least 5% better on the old one than the new one. If you disagree I'd bet about that! Since your objection only really seems to make any sense if we consider they're actually sampled from a similar distribution (which is to say, they're similarly hard problems, for AIs), and I expect that to not be the case, I'm unconvinced, but if I'm wrong about it then your point is worth making even though it shouldn't imo change the resolution criterion now settled on.
also again, please remember for this market and all subsequent ones, that on manifold the description always wins over the title, so really make sure to bet based on the description if there exists one. I'm sorry if you feel tricked or cheated about that.
@Bayesian I am not sure I follow your idea for a new market. The spirit of ARC, as I understand it, is to provide grid images that the average intelligent person can solve but which are hard for ML algorithms. As long as you can provide such images (and verify that humans can solve them), you are 'sampling from the distribution.'
The spirit of ARC is decidedly not to (implicitly) encode a certain number of heuristics into your model, but this is what inadvertently happens when you have data leakage (for example, from breaking into Chollet's apartment, or from a huge number of tries on the 'private' test set.) As someone with a background in ML/AI @JacobPfau should presumably understand this, which makes it worse.
I also know that description is more important than the title. What I am saying is that the description is pretty unambiguous though: '>=85% performance on Chollet's abstraction and reasoning corpus, private set,' and I think the way you guys are now reading it is a reinterpretation, especially if read alongside the original title, which makes it clear that the task is to achieve 85% on private samples (no leakage, which means continual updates are necessary). The example with the break-in makes it pretty clear why insisting on using the 'original' samples is not in line with the spirit of the market.
@CalibratedNeutral Leakage for huge labs is not a very big issue because most of them (including anthropic, openai and deepmind) don't want data leakage destroying the utility of the benchmarks. And no, the fact that you are sampling from the distribution is not implied by the fact that you're generating grid images that the avg intelligent person can solve but which are hard for ML algorithms. I can provide a counterexample.
Suppose you have a set of 100 questions that are just hard enough that intelligent humans can solve them but they are slightly too hard for ML algorithms.
Suppose you have a second set of 100 questions, which are hardish for intelligent humans, but extremely hard for ML algorithms.
Both of these sets of questions fit the "spirit of ARC", but they are not part of the same distribution because their degree of difficulty for an ML algorithm that wasn't trained on either one will be very different. It is important to know whether, assuming no leakage, an ML algorithm would do as well on the previous set of questions as the new set of questions. If they do noticeably worse on the second set, and it's an AI lab prestigious enough that they will make great effort to not get data leakage problems (so we assume there is none), then the second set is what I and most people would consider "harder for ML algorithms". I am proposing a market that checks whether the new set of questions is merely new questions that are as hard for ML algorithms that haven't fallen prey to data leakage, or if they are just new questions that are harder than the previous questions. does that make more sense?
@Bayesian Leakage is in fact a big issue for huge labs and, in particular, OpenAI because they are incentivized to achieve high scores to get publicity and money. They are primarily a commercial company, not a research lab or academic institution, and even the latter are known to game benchmarks, as everyone in the field knows, even though few admit it.
The distribution is, in fact, defined exactly the way I wrote. Your counterexample falls short because, by trying to improve your score on any finite sample from a distribution, you are inadvertently starting to overfit on that sample. This is the reason for having a test set (which you literally hold out) in the first place. You want to report a final estimate of your model's performance on new samples from the underlying distribution. You no longer have an unbiased estimate once you start to "improve" your model based on the score you have seen.
If you assume that Chollet created thousands of more grid images/tasks he didn’t tell us about, which are easily solvable by humans, then the latter part must be the defining criterion for what it means for a grid image to come from "the ARC distribution." Once you get access to your score on any finite "test set" and start to tweak your algorithm to improve that score, you will get better performance on that set, and it should no longer be considered a test set in the truest sense. Improving your algorithm includes things like architecture choice and even more subtle notions like the way you think about the problem, by the way, so the impartial ML algorithm you imagine for your other question does not exist. "No free lunch" also comes to mind here.
In the end, you have to trust that the underlying distribution makes conceptual sense, and we should assume it does because the creators use real people to try and solve the tasks. If Chollet suddenly added tasks that were way harder to solve for humans, then I would agree with you that they could no longer be considered to come from the same distribution. To try and define the distribution in terms of algorithms having a harder time on newly sampled data is thinking about the problem in the wrong way.
To clarify, for this market to resolve yes the 85% needs to go through the limited compute Kaggle setup right as well as being open source right? @JacobPfau https://x.com/fchollet/status/1870170897283526723
@MalachiteEagle Chollet wrote "It scores 75.7% on the semi-private eval in low-compute mode (for $20 per task in compute ) and 87.5% in high-compute mode (thousands of $ per task)" - what is a semi-private dataset and why does it satisfy resolution criteria (">=85% performance on Chollet's abstraction and reasoning corpus, private set")?
@Bayesian ahh right open source / open weights criteria. Might be a good idea to mention that in the description
@Metastable I'm guessing the semi-private dataset is called that because they're supposedly only sharing it with OpenAI through the API for o3 or something
@MalachiteEagle the competition is on the private set, not the semi private set. Semi-private set is used for models that work through API to prevent leakage of the private questions. In addition, the competition has compute requirements which are likely way exceeded by o3.
@na_pewno (turns out the low compute score - 75.7% on the semi-private set - is within the compute requirements - https://www.youtube.com/watch?v=SKBG1sqdyIU 7:40)