
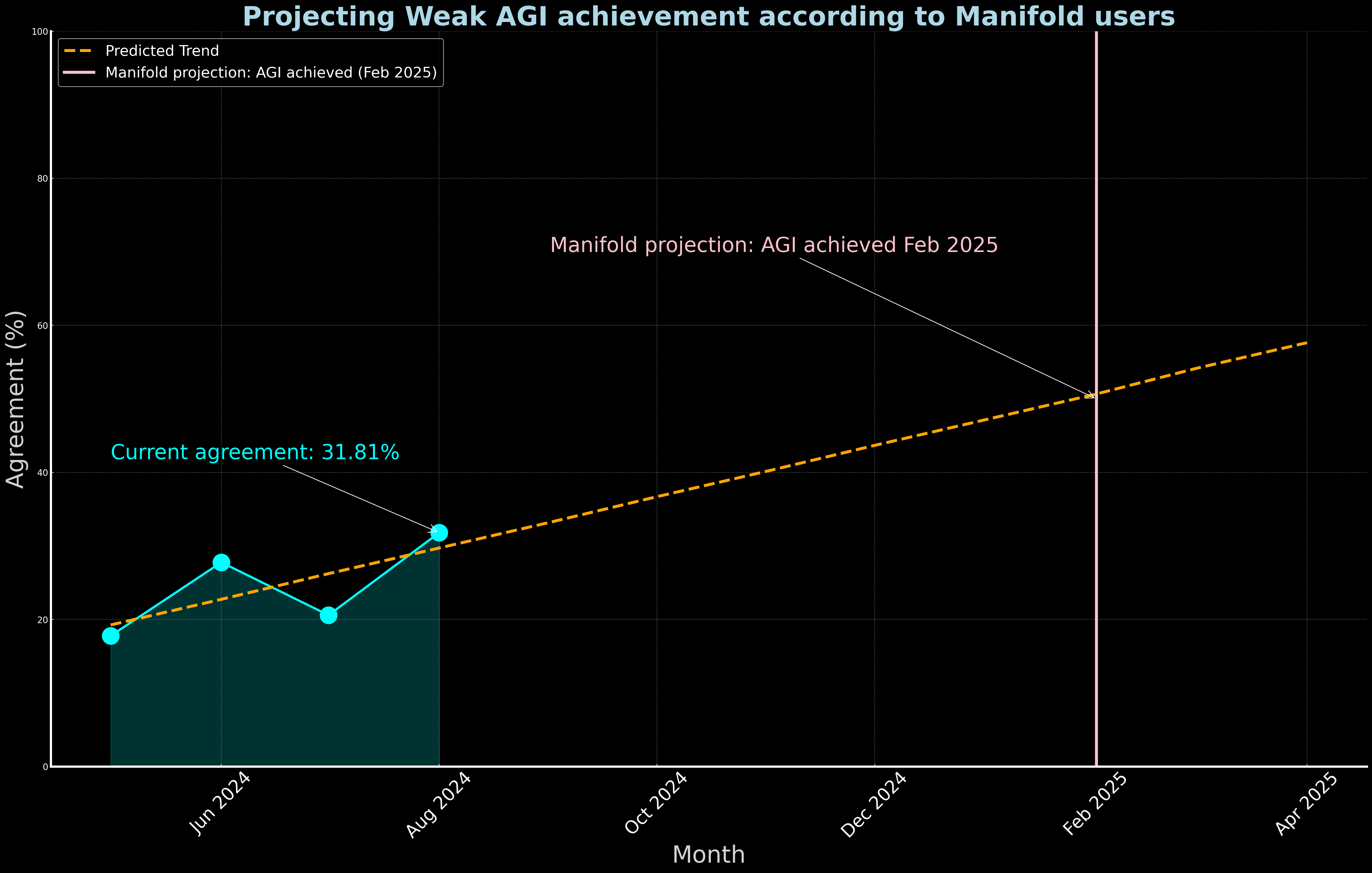
Every month, Manifold users are invited to vote on polls that ask whether humans have achieved weak AGI. In the polls, the definition of "artificial general intelligence" is not provided except to state that manipulation of the physical world is not required for "weak" AGI. The polls open on the 23rd of each month and close seven days later.
Currently, approximately one third of Manifold respondents believe that weak AGI has been achieved.
The trendline projects that Manifold will declare the achievement of weak AGI with half of users in agreement during February 2025. Excluding "no opinion" respondents, will Manifold make that declaration with one of the monthly polls during or before that date?
RELATED:
@ProjectVictory I believe that linear is probably not the appropriate regression to use when discussing technological development.
The polls are becoming stronger with each passing month, because they have more data. I am most confident in the highest (latest) result because it had the most respondents.
I lean towards YES in this market and will bet that way. I already answer YES to the polls because it's absurd to believe that Claude 3.5 Sonnet is dumber than 99% of the world. I think the correct projection is more exponential, because we are discussing technology, and this will probably resolve in December, not February. However, there were similar questions like the following:
/SteveSokolowski/will-at-least-20-of-manifold-respon
where users bet down the market very close to NO first - because people always underestimate technological progress. Even "AI experts" do. I expect that there will be a lot of users who bet this market down below 20%, so I will wait and then start bidding YES when that occurs.
@SteveSokolowski linear is the wrong not because of the technological development or the different weights of the datapoints.
It is wrong because on the probability plot we should always search for a function, which can never cross 100% (like sigmoid-type functions).
But I understand that with only 4 points nothing better than linear can be formed, and can be said to be some zoomed in other function.
@SteveSokolowski I think it's purely a semantics argument we are having here. I voted no on polls because being more useful than the 1% dumbest person isn't my definition of AGI. I would definitely prefer to work alongside an LLM rather than the dumbest person I know, but I don't find this a useful benchmark.
Also, if "people always underestimate technological progress", doesn't that mean the polls should still resolve no, despite AGI being achieved?
@ProjectVictory I answer the polls YES because I believe the definition of AGI, as far back as when I was trying to figure out neural networks in 2003, was always when computers would be smarter than the average human.
LLMs are undoubtedly without question smarter than the average human. Humans make mistakes with lies, just like LLMs do; the type of mistakes are different. I would go so far that they are smarter than 99% of humans at this point.
But the point of the polls is to see when society accepts that AGI has been achieved, which is why I never define the term or comment on it in the polls themselves.
@SteveSokolowski My personal experience is very different. I don't consider myself to be in the 1% smartest people, but talking with LLMs I often find myself correcting it, rather than being corrected myself.
@ProjectVictory I think it depends on the topic you are conversing about.
One thing I've found that LLMs are better at compared to any other thing they can do is designing models. They understand themselves well and can spit out code that runs on the first try and significantly improves the accuracy of models. I've been surprised many times at how I look at the code and make changes to it, and then later end up reverting because it turns out that the LLM was getting at something that I had never considered before.
It may be that LLMs are weak in very specific circumstances that you use frequently. For model design, they are better than almost any human at this point.
@SteveSokolowski being better than you at this is not strong evidence of being better than any human alive. There are people actually doing research on model architecture and design who have thousands of citations and have contributed the theoretical basis for the progress in these systems.
Anything you are doing which already has lots of examples in the training set isn’t particularly novel and I don’t see anything on google scholar under your name in this field. LLMs are extremely useful tools but to say they are better than every human at designing ML models requires ignorance of what the people at the top of the field are actually capable of.
What you are really saying is that these companies have lots of code written by very clever people for working with these types of systems and, while it’s not all public or easily searchable, a lot of that code was used during training. As a result, their cleverness has been encoded in the weights and you can now benefit from it without necessarily knowing the sources.
That’s not that surprising when you consider that these models are made by ML experts with easy access to lots of LLM code.
@LiamZ You're correct about that - even if I were a world-renowned model expert, I wouldn't want my name in any publications, as that probably hurts my models' ability to make money in the stock market.
Remember, I didn't say they were better than any human in the text above, so I would be careful about how you word the response. I do believe that they are better than 99% of humans at most tasks - particularly model design. And, whatever the reason for that, they do enable people to build great models easily.
You did say better than almost any human. The bit about not wanting your name in publications is disconnected from reality. And most better at most tasks? GPT 4o can’t even consistently draw me in tic-tac-toe.
The vast majority of tasks humans deal with day to day require a more coherent world model than we’ve managed to encode in models so far, I wouldn’t trust any model to solve the series of problems required when I survey the contents of my fridge, walk out of my house, drive to a grocery store, pick out items taking into account the dietary restrictions and preferences of my family based on what is available, fresh, on sale, etc., pic up each item, place it in my cart, navigate through the store, and then return home.
One can see how each step in a problem like this potentially tractable and that’s a single daily task which actually does have a large amount of training data available for each step so it’s reasonable to expect these to be eventually addressed. This is a task which does not require high intellectual engagement or creativity and has had active effort costing insane amounts of money on each step yet current models are outclassed by the average 16 year old at completing this task.
@LiamZ Isn't this the problem that's well known where things that are easy for humans are difficult for computers and things that are difficult for computers are easy for humans?
Remember that these polls are surveying "weak" AGI, although I do think that the context window of Claude 3.5 Sonnet is large enough that you could have it produce the grocery list given several camera angles of the fridge's contents, what you need in total, and the dietary restrictions, and have it forget fewer items than the average human.
I'm with you in expecting that one of the first, if not the first, thing that models will become superintelligent at is designing models because there is probably more training data available on model design, and it only involves computer programming, compared to any other subject.
@SteveSokolowski A few replies above you claimed, “LLMs are undoubtedly without question smarter than the average human.”
To be explicit, I believe this to be a category error mixing up memorization of the output of human intelligence with intelligence itself. Good memorization leads to the same outcomes as intelligence under circumstances for which training data exists. Yet, it fails when faced with novelty.
LLMs are useful because a lot of things we do can be well solved by interpolation from the creation of other humans and this function approximation gives us a meaningfully useful tool for performing that interpolation. Yet, the minute one steps outside the training data, the solution region is no longer mapped and novel solutions requiring intelligence are required.
One need only make a few modificiations to an existing riddle to see spectacular failure of these systems to encode an ability to reason rather than memorize examples of reasoning.